For AI to create value for business and society, the data that trains and feeds models must be trustworthy.
Trust in data starts with transparency into provenance; assessing where data comes from, how it’s created, and whether it can be used, legally. Yet the ecosystem needs a common language to provide that transparency.
This is why we developed the first cross-industry data provenance standards.
Provenance matters. Understanding the sources of food, water, medicine, capital is expected and essential in our society to gauge quality and trust. The same is now needed for the fuel of our increasingly knowledge- and AI-centric world: data.
The standards were derived from use cases across 15 different industries that outlined the data provenance challenges faced within business today. These were then synthesized, refined, and validated by a team of practitioners: chief technology officers, chief data officers, and leaders in data governance, data acquisition, data quality, privacy, legal and compliance. These standards were not developed to inform regulation.
The Data Provenance Standards were designed for adoption across business, which is why they were built with three aspects in mind: Increasing business value, ease of implementation and complying with new and emerging regulation.
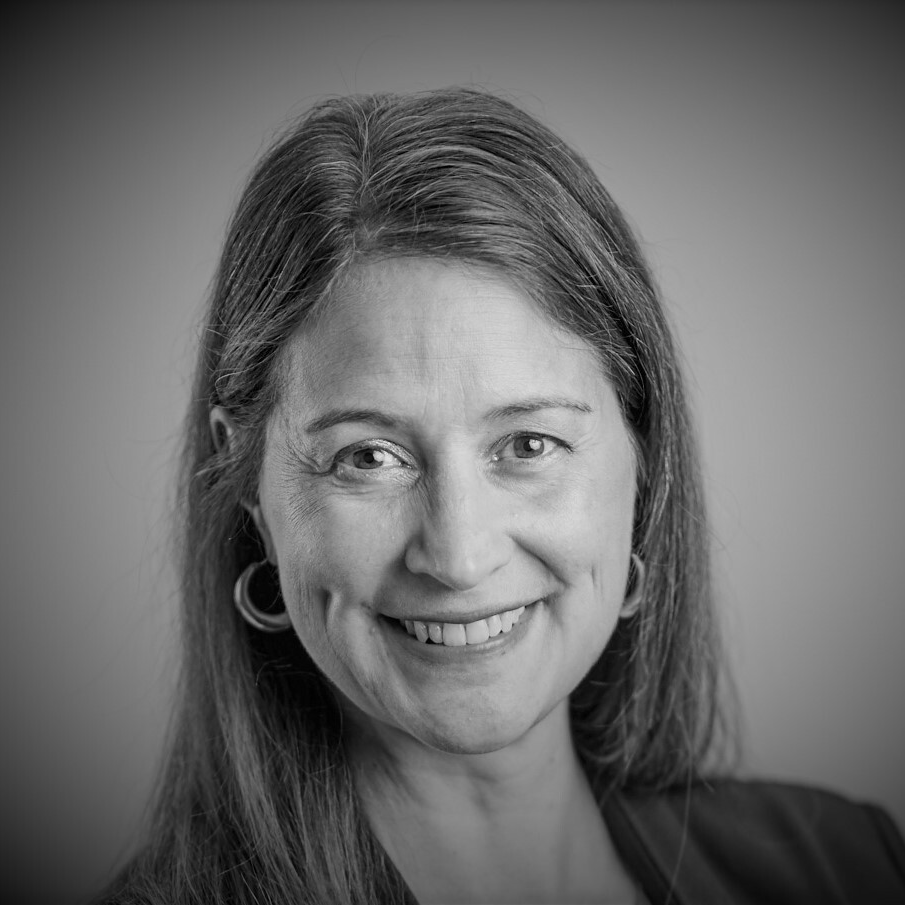
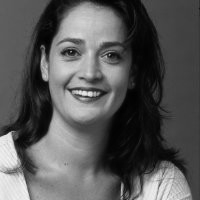
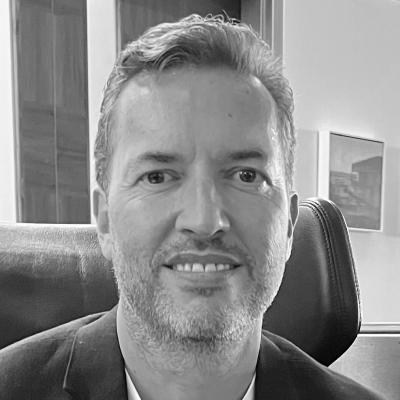
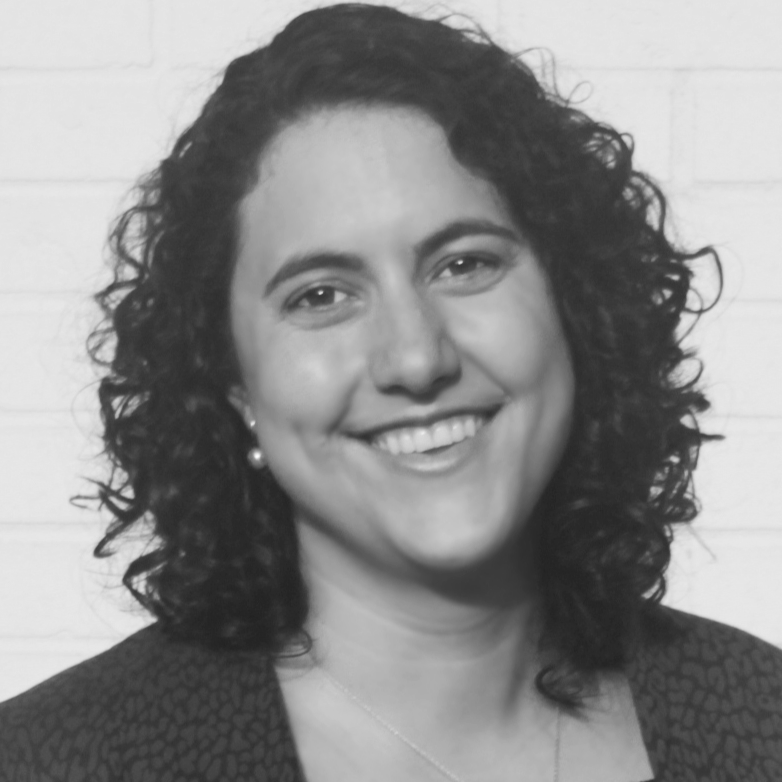
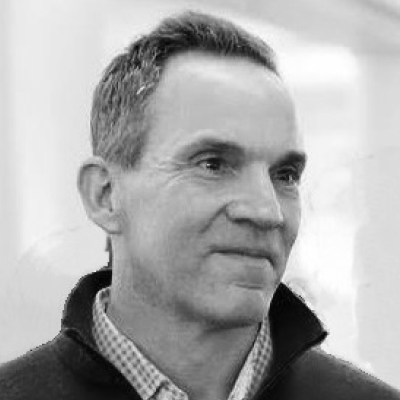
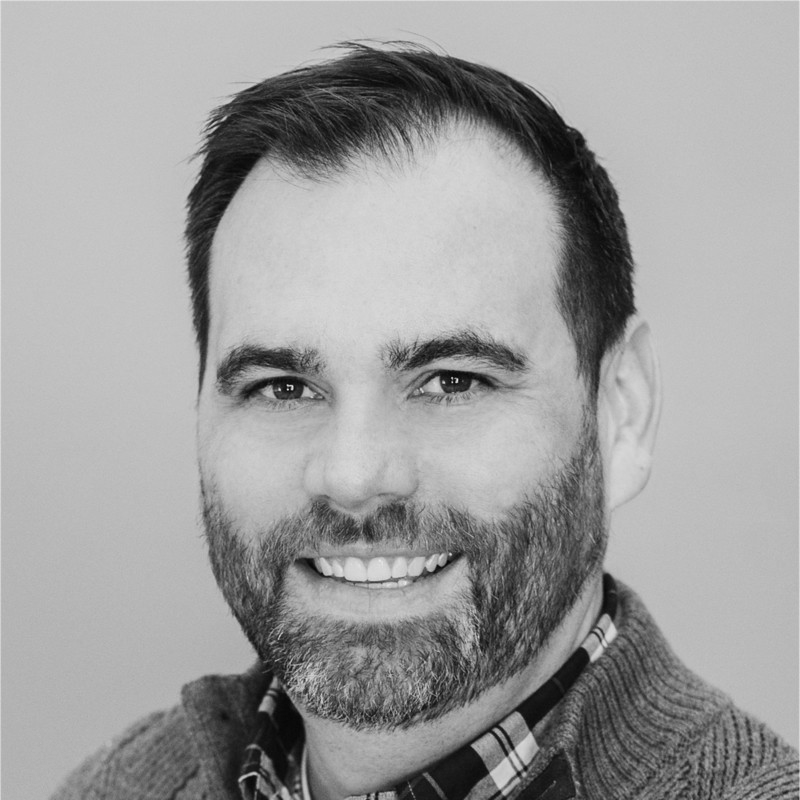
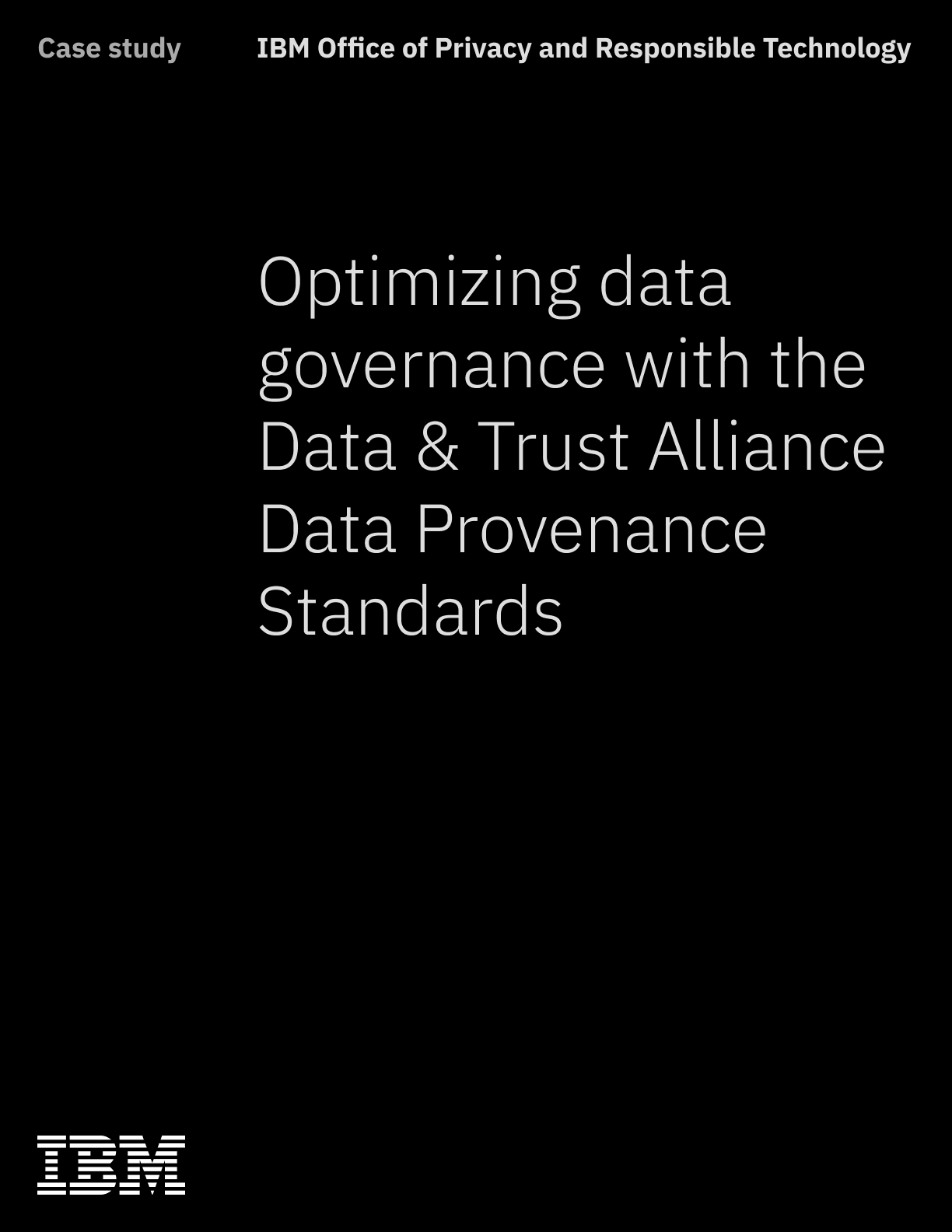
In early 2024, IBM tested the standards as part of their clearance process for datasets used to train foundational models. They saw increases in both efficiency (time for clearance) and overall data quality.
Read the IBM case study ->The Data Provenance Standards consist of 22 metadata fields, grouped into 3 standards: Source, Provenance, and Use.
Standards version used
Dataset title/name
Unique metadata identifier
Metadata location (unique URL of the current dataset)
Dataset issuer
Description of the dataset
Source metadata for dataset
Source (if different from Issuer)
Data origin geography
Dataset issue date
Date of previously issued version of the dataset (if applicable)
Range of dates for data generation
Method
Data format
Confidentiality classification
Consent documentation location
Privacy enhancing technologies (PETs) or tools applied?
Data processing geography inclusion/exclusion
Data storage geography inclusion/exclusion
License to use
Intended data use
Proprietary data presence
Download Use Case Scenarios to understand how the standards inform decision making across different scenarios
Access the Data Provenance Standards metadata generator to create and download standardized metadata files in JSON, CSV, or CML format to meet the Data Provenance Standards and facilitate data sharing
Visit the Technical Resource Center on GitHub for technical standards specifications, code snippets, and other implementation assets
Join the Community of Practice
Request changes to the Data Provenance Standards using the Change Request Form
Data provenance standards establish criteria for documenting the origin and lifecycle of data, which includes details on how data is collected and how it may be used. This transparency is crucial for driving accuracy, reliability and trustworthiness of data, across all industries.
The Data Provenance Standards were co-developed by 19 different member organizations at the Data & Trust Alliance (D&TA), which represents some of the most significant users of data and AI across industries today. The group was made up of chief technology officers, chief data officers and leaders in data acquisition, data governance, data strategy, data quality, legal and compliance. The goal was to establish a uniform approach to increasing transparency in datasets, thus increasing the integrity of and trust in data and the AI that it feeds.
Yes, they are designed to apply to all industries. While we believe they are especially beneficial for sectors like healthcare, finance and technology, any industry that handles data and uses it for AI can benefit from implementing these standards.
All companies, regardless of size, are encouraged to adopt these standards. Implementing them may not only strengthen trust in your data integrity within the broader data ecosystem, but also can signal that your business is a leader in data transparency and reliability efforts. Adoption can serve as a competitive advantage, showcasing your commitment to best practices in data management and increasing the value of your data and AI in the marketplace.
Our standards underwent testing through diverse scenarios, including traditional data acquisition, synthetic data tracking in AdTech, and governance for large language models. This cross-industry approach, spanning companies of all sizes, was complemented by validation from industry experts and governance groups. This inclusive process ensured our standards quickly evolved to meet a broad range of stakeholder needs, making them robust and relevant.
Adopting these standards enhances data transparency, which produces a range of business value. At minimum, the transparency can lead to efficiency and cost savings by decreasing time to data acquisition, cleanup and pre-processing. It also improves quality assurance and security, which are foundational to innovating and building new value with data. Finally, this data transparency can help organizations seeking to comply with existing and emerging data protection laws and provisions in AI regulation. In sum, these standards are designed to increase trust in data use and sharing between organizations and with consumers.
By providing a clear history of data provenance and its appropriate use, these standards help in auditing and monitoring data use, thus supporting data security and regulatory compliance efforts.
For data suppliers, the primary technical requirement is a website where you can publish metadata, which can be captured either through our web interface or a standalone spreadsheet. As a data consumer, there is no need for any technical infrastructure to read the metadata. However, if you intend to pass data downstream to other consumers, you will need the same infrastructure as a data producer, including capabilities for data logging and tracking to ensure compliance and maintain the integrity of the data provenance.
No specific technologies are required, but for future-proofing, you might consider making systems capable of integrating with APIs and services that support metadata management and audit trails.
These standards complement data protection laws by enhancing the ability to test compliance through clear data lineage and permitted use, including data storage and processing requirements and documentation. They do not guarantee compliance with any particular data protection law.
There are no legal requirements for adopting these standards. However, adoption can form a part of data privacy compliance efforts.
The initial step towards adopting the standards varies by organization, but generally starts with understanding your current position relative to the new standards. Here’s a streamlined approach to get started:
Assessment: Evaluate your existing data management practices to identify discrepancies and areas for improvement compared to the standards.
Engagement and socialization: Host a community of practice meeting to discuss the standards. If you’d like to invite insights from Data and Trust Alliance (D&TA), get in touch and we can attend your event. Or consider conducting an interview with an executive sponsor in your organization to discuss the value and adoption of the standards during an all-hands or company-wide meeting.
Integration and implementation:
Incorporate the standards into key business processes, such as those led by Project Management Offices (PMO) and Data Governance Boards.
Develop and disseminate internal policies that align with these standards.
Adjust procurement strategies to include standards compliance.
Budget for the necessary resources to adopt the standards.
Utilize internal communication channels like Slack to keep discussions about standards active.
Deploy as a proof of concept and generate supporting metrics:
Launch a proof of concept with a data provider to build confidence within your team or broader organization.
Incorporate the standards into audit and compliance routines.
Apply the standards directly to your data systems for enhanced transparency and trust.
Analyze how your data catalog aligns with the D&TA standards.
Integrate these standards into governance tools like Collibra, Informatica, or Databricks.
Define and implement metrics to monitor adherence to these standards, including third-party compliance.
The timeline will vary, but we estimate that it could generally range from a few months to more than a year, depending on the size of your organization and the complexity of your data systems.
Resources may include, among others, data, analytics and IT staff for system integration, legal and compliance teams for regulatory alignment, and training personnel.
Challenges include aligning existing systems with the standards, training employees, and managing the initial costs.
Success can be measured by improved compliance audit results, reduced audit issues, increased efficiency and efficacy in assessing whether data is fit for purpose, as well as enhanced data partner trust.
Key metrics include the number of data corrections needed, audit trail completeness and compliance audit results.
Companies can join the D&TA Community of Practice on LinkedIn and participate in related community events and discussions. We foster our community of adopters and encourage everyone to share experiences, best practices and support. Engagement provides networking opportunities, insights into effective implementation strategies and peer support.
Please visit our Community of Practice on LinkedIn. This will move over to the OASIS-supported community over the next few months. Please note that views expressed by adopters in the D&TA Community of Practice represent the views and opinions of the views of such adopters, and do not necessarily represent the views or opinions of the D&TA, and the D&TA makes no representations or warranties of any kind with respect to those views.
Yes, the D&TA facilitates a community where adopters can share experiences, best practices, and support. Please visit the D&TA Community of Practice on LinkedIn.
The standards will be updated through feedback from an open practitioner community, managed by the standards body OASIS. The community will be a place for use cases, feedback, troubleshooting, and the eventual evolution of the standards. At the time of publications, we expect that the current v1.0.0 will be stable for at least 12-18 months.